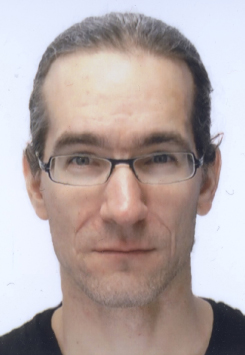
Christoph D. Dahl
- Animal behaviour
- Electrophysiology/extracellular recordings
- Computational modelling
- Machine learning
My research programmes focus on
Computational ethology
Computational ethology is the interdisciplinary research domain that addresses the lack of instrumental methods in ethology by introducing tools originating in mathematics and computer sciences, in particular in artificial intelligence and machine learning. The defined goals of computational ethology are to (a) detect predefined behaviours in freely moving animals and (b) discover novel behavioural patterns and new behaviours (Wiltschko et al., 2015; Dahl, Wyss, Zuberbühler, & Bachmann, 2018).
1. Christoph D Dahl, Elodie Ferrando, and Klaus Zuberbühler. An information-theory approach to geometry for animal groups. bioRxiv, page 839548, 2019.
2. Christoph D Dahl, Christa Wyss, Klaus Zuberbühler, and Iris Bachmann. Social information in equine movement gestalts. Animal Cognition, 21(4):583–594, 2018.
Higher-order cognition in insects
The goal in this research programme, is to (a) describe similarities (and dissimilarities) of insect and mammalian sensory systems (Borst & Helmstaedter, 2015; Wilson, 2013), particularly in combination to higher-cognitive processes, to (b) understand the neuronal circuitries of internal representation and to (c) pursue these objectives in a virtual and actual environment; the latter providing free animal behaviour partly in interaction with conspecifics. The study of insect cognition and cognitive computation is in very early stages, however, providing a unique window into the function of neuronal mechanisms and circuitries underlying cognition due to a samller and ”simpler” brain.
Social interaction and group dynamics in zebrafish
A useful computationally and quantitative valid description of the behavioural dynamics in groups of zebra fish during learning tasks is essential for the subsequent establishment of a link between social behaviour and neural activations using modern neuroscience methods. This data-driven research approach for characterizing social interaction behaviour in groups of animals is only just beginning, but already led to very promising results. For instance, by tracking social interaction in groups of mice a new high-order structure was found [Shemesh et al., 2013]. In particular, it was found by tracking the exact movement trajectories of a group of mice in a semi-natural environment over a long time that the statistics of the spatial configuration of the mice over time could not explain by a model only relying on pairwise correlations. Instead higher order terms had to be taken into account to explain the data hinting at a more complicated social interaction structure as previously thought. The goal of this research is to establish a similar data-driven approach to characterize social interactions in zebrafish. Moreover, we are interested in the changes of the dynamics of the interaction structure and the characterization of the flow of knowledge (e.g. information about a reward source) from one individual to others while the group performs a social learning task.
Feature extraction and selection for animal vocalizations – a methodological comparison
Feature extraction is a critical step in the processing of highly-dimensional data sets, as it reduces the complexity to a minimally redundant and maximally informative subset, hence reducing the memory load and computation power. Deriving informative values, so-called feature dimensions, requires knowledge about the nature of data. In traditional domains, such as primatology and animal behaviour, little has changed regarding the extraction of values from audio (and behavioural) recordings. Estimated 20’000 peer-reviewed studies relied on manual and experience-dependent extraction of basic audio properties, such as formants. Trends from speech recognition research, namely the extraction of features that account for the fact that vocalizations generated by an animal are filtered by the shape of the vocal tract, slowly enter the fields of primatology and animal behaviour. A major open question in primatology is to what extent primate vocalizations are meaningful units of communication.
1. Guillaume Dezecache, Klaus Zuberbüler, Marina Davila-Ross, and Christoph D Dahl. Machine learning reveals adaptive maternal responses to infant distress calls in wild chimpanzees. bioRxiv, page 835827, 2019.
2. Guillaume Dezecache, Klaus Zuberbühler, Marina Davila-Ross, and Christoph D Dahl. Early vocal production and functional flexibility in wild infant chimpanzees. bioRxiv, page 848770, 2019.
3. Guillaume Dezecache, Klaus Zuberbuhler, Marina Davila-Ross, and Christoph D Dahl. The evolution of crying: Distress calls and infants’ needs in wild chimpanzees. In Folia Primatologia, volume 88, pages 120–121, 2017.
4. Pawel Fedurek, Klaus Zuberbühler, and Christoph D Dahl. Sequential information in a great ape utterance. Scientific Reports, 6:38226, 2016.